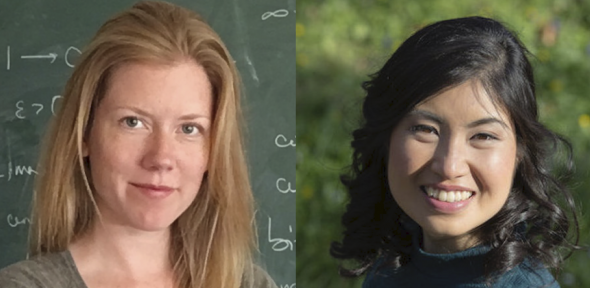
Two of the five winners of the 2023 Philip Leverhulme Prizes for Mathematics and Statistics are members of DPMMS. Professor Po-Ling Loh and Professor Holly Krieger have each been awarded a prestigious £100,000 prize.
The prizes are awarded annually by the Leverhulme Trust to recognise and celebrate the achievements of outstanding researchers whose work has already attracted international recognition and whose future careers are exceptionally promising. The prizes can be used for any purpose to advance the winners’ research.
"I am delighted that Holly Krieger and Po-Ling Loh have both received a Leverhulme Prize in well-earned recognition of their outstanding work," said Professor Ivan Smith, Head of the Department of Pure Mathematics and Mathematical Statistics. "I hope the Prize will help them build new and strengthen existing collaborations."
Energising the intersection of number theory and complex dynamics
Professor Holly Krieger has been recognised for her work on the interaction between number theory and complex dynamics.
Holly Krieger works in the field of arithmetic dynamics. "Arithmetic dynamics is exactly what you get when you take the two words apart," Krieger explained in our interview when she won the 2020 Whitehead Prize. "Arithmetic relates to those very simple notions you learn about at school: counting, integers, prime numbers and so on. These are the fundamental building blocks of what we think of as abstract mathematics." The term ‘dynamics’ refers to systems that evolve over time. These can often be quite easily described mathematically, but can still exhibit incredibly complex behaviour in the long run.
Krieger was partly drawn to working in arithmetic dynamics by the age-old lure of number theory, whose seemingly simple questions often turn out to be fiendishly hard to prove. "I like the simplicity of the questions, combined with the difficulty and depth of the techniques," she says.
But while number theory is old, arithmetic dynamics is new. "Arithmetic dynamics is a relatively young field, so it has a lot of energy. In the 1990s it was realised that there's a very deep connection [between the theory of dynamical systems] and hard questions in number theory. The question was, 'what can we do with this analogy; is there power there?' This led to an entirely new way of studying dynamical systems. It's that innovation that I liked."
You can read more about Holly Krieger's work in our feature article Dynamic Numbers.
Taking statistics into new dimensions
Professor Po-Ling Loh has been awarded the prize for her work on theoretical statistics, spanning topics including high-dimensional statistics, robustness, optimisation, and privacy, with machine learning applications.
High-dimensional statistics is an exciting new field. In the last 15 to 20 years fields such as genomics have produced data sets that are very different to those traditionally analysed in statistics. "If you sequence a genome then there are tens of thousands of genes that you are interested in, but the number of people you are sampling is only in the hundreds," explained Loh when we interviewed her last year. "When you have these two dimensions playing off against each other – the number of parameters [you are measuring] and the number of samples – you need to do some more careful analysis to understand what is going on."
One of the areas Loh has worked on is non-convex optimisation for high-dimensional statistics. Optimisation involves trying to find the minimum (or maximum) of a function, something that is straightforward for convex functions. However, the functions that were arising from the types of problems that Loh was interested in were not convex. Instead of being a bowl-shape, like a convex function, they might have local minima – where a flat place in the function might not actually mean that it's the very lowest point.
Loh realised non-convex optimisation is also something that arises in an area called robust statistics, which studies data that may have been randomly contaminated. The contamination might have come from some systematic source (say from accidental measurement errors) or the data could have been intentionally contaminated by an adversary. This area became popular in the early 1960s and has led to statistical methods that perform well in the face of contaminated data, outliers or with data that doesn't fit an ideal distribution.
There's been an influx of interest in robust statistics with the development of machine learning. "People knew that these machine learning algorithms were not robust, and the question was how can we make them more robust,” said Loh. "That's what I'm currently excited about – studying more robust algorithms and rigorously showing that these methods perform well even when you might be in this high-dimensional statistical setting."