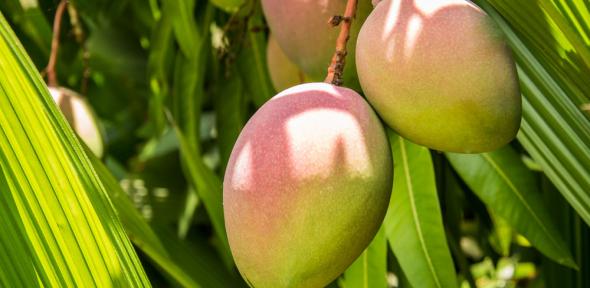
Revolutionary machine learning techniques are being developed by researchers from the Department of Applied Mathematics and Theoretical Physics (DAMTP) to support forest conservation in India.
The aim of the INTEGRAL project is to develop algorithms that can recognise species of trees in images taken from the air without any human input. These would be able to process vastly more images than any team of humans ever could, and they could be used to assess biodiversity of forests in regions across the globe. Understanding the composition of forests is important because of the role they play in climate change—storing carbon—and because they can provide a home for an abundance of other species.
Although forest ecology is an important focus of the INTEGRAL project most of the researchers involved are mathematicians. That's because the algorithms needed to assess biodiversity on a large scale push the boundaries of state-of-the-art artificial intelligence. New mathematical methods are required to develop them.
"It's a very new technique we are applying to real time data sets," says INTEGRAL member Debmita Bandyopadhyay of DAMTP. "[This is] is a huge challenge where a lot of misinterpretation can take place: the forests in India are mixed forests so from one [image] pixel to the other species can change. We are facing challenges, but we are reaching there."
It's a very new technique we are applying to real time data sets. We are facing challenges, but we are reaching there. Debmita Bandyopadhyay
Mapping the world
The work of INTEGRAL builds on DAMTP's existing expertise in image analysis, and will be useful in a wider context too. Modern life produces an abundance of images, taken by anything from traffic cameras to satellites, containing information that could never be extracted by humans alone.
"Remote sensing, [understanding an area through images], is a major means for mapping our world," says Carola-Bibiane Schönlieb, Professor of Applied Mathematics at DAMTP and co-lead of INTEGRAL. "This data by itself is useless if we do not have the means to analyse it, to extract the information from it that we are interested in."
The mathematical techniques that make remote sensing possible can be used in any context, whether the object you'd like to recognise is a tree in an aerial photograph, a vehicle in an image from a traffic camera, or a tumour in a medical scan. This synergy is something the INTEGRAL project exploits. While India's ever expanding cities threaten its forests, the vast amounts of traffic within those cities also threaten human health. Another strand of the project is to use data from traffic cameras to assess the composition of traffic in those cities to inform the decisions of planning authorities — you can read more about the traffic part of the project in this article.
Teaching machines to learn
The kind of artificial intelligence being employed by the INTEGRAL team is called machine learning. This involves an algorithm learning to spot patterns in a data set that correspond to structures hiding inside that data set. In the case of remote sensing, the data sets are images (which, in a computer, are represented as arrays of numbers) and the patterns indicate whether the image depicts a particular type of tree or, in the case of traffic, a particular vehicle.
The trouble with machine learning in its simplest form is that it needs to learn from a set of training data which is already labelled with the correct answer, for example whether it's a mango tree or a palm tree. But the task of providing such annotated data alone already requires a lot of expensive human input.
"State of the art AI approaches come with a price," explains Schönlieb. "They need a lot of very high quality annotated data to be trained on. In applications where we are dealing with real data such annotation is very costly and time consuming to obtain, either because expert knowledge is required to do the annotations, and/or because there is a lot of manual work involved in collecting the data on the ground or sitting in front of a computer doing the annotations. This is where the mathematical motivation of INTEGRAL comes in."
To deal with this challenge the INTEGRAL team are developing so-called semi-supervised learning techniques. Here algorithms make maximal use of information inherent in the training data to make do with a much smaller amount of annotated data. It seems like magic, but it does work.
Connecting the world
A key part of the INTEGRAL project is the collaboration between India and the UK. Apart from scientists and mathematicians at the University of Cambridge, the project comprises a range of organisations, including the environmental advisory group IORA Ecological Solutions, Forest Survey of India, Indian Institute of Technology Delhi, and the Indian technology company Kritikal Solutions.
Experts on the ground in India play an important role. "To train the AI [still requires] some human [input]," says Saurabh Pandey from KritiKal Solutions. "We can say whether the red patch you see [in an image] is Mango trees, or something else entirely. The sensors will tell you the colour of a particular pattern and the data that we collect will tell you that the pattern belongs to a particular species."
"We're at a very exciting moment, where we have the field data, the [images] from aircraft , and Debmita working very hard to test the methods we have developed on these data sets," says David Coomes of the Department of Plant Sciences at the University of Cambridge and co-lead of INTEGRAL.
"Once we have got these classifications working there are all sorts of opportunities to apply them elsewhere. The UN has declared a decade of forest restoration around the world, so there's a huge appetite for these species maps, which are based on INTEGRAL work."
You can read a more machine learning and semi-supervised learning on Plus magazine, and hear from the INTEGRAL team themselves in this Plus podcast.