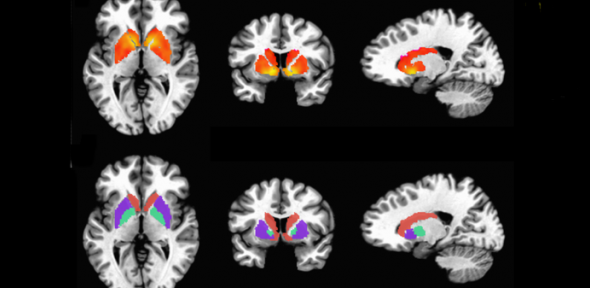
Could artificial intelligence support medical doctors in their work? The Cambridge Mathematics of Information in Healthcare Hub (CMIH) aims to make this vision a reality.
Part of a doctor's task is to process data — blood counts, MIR, CAT or X-ray images, and results from other diagnostic tests, for example. Since data processing is something that computers are particularly good at, the idea that they should be able to help in this context seems natural.
With the rise of machine learning, the most successful recent development in AI, there's hope that computers will be able to do much more than basic data crunching. Algorithms may become sophisticated enough to measure the exact dimensions of a tumour from an MRI scan — something that takes a human hours to do — or integrate different pieces of information to suggest difficult diagnoses, for example for Alzheimer's disease. They may also open the door to a more personalised form of medicine, where treatment plans can be carefully tailored to an individual patient based on everything that is known about them — including, for example, complex genetic information.
The Cambridge Mathematics of Information in Healthcare Hub (CMIH) has been set up to make these ideas a reality. The Hub is being co-led by Carola-Bibiane Schönlieb, Professor of Applied Mathematics at the Department for Applied Mathematics and Theoretical Physics, and John Aston, Harding Professor of Statistics in Public Life at the Department of Pure Mathematics and Mathematical Statistics, with James Rudd of the Department of Medicine in Cambridge acting as clinical lead. The aim is not for artificial intelligence to replace doctors, but to work with them. The results will be open source tools that are ready to use in hospitals and other clinical settings, freely available on an online platform.
Exciting challenges
It's a pioneering vision that comes with many challenges. First and foremost, tools will need to be extremely accurate. There’s no room for errors when people’s health and lives are at stake. Then there's the sheer complexity of the data involved. A person’s gut, for example, is a dynamic and elastic object that will look different every time you take a picture of it. Spotting an abnormality within that picture can be hard for a human, and is extremely tricky even for the most advanced image analysis algorithm. Some diseases, such as Alzheimer's, are diagnosed based on a board range of information, including a person's way of speaking. And while automated speech recognition is just about good enough for Siri or Google Voice, it’s not yet accurate enough for medical purposes. In addition to dealing with complex data from a single source, such as images or speech, clinical tools should also be able to work with combinations of them.
More challenges come from the way in which machine learning works. It involves algorithms "learning" how to spot patterns in data, such as MRI images of a person's gut, that indicate there are certain structures within the data, such as a tumour. To do the learning, the algorithms require training data (images in our example) for which the answer (tumour, yes or no) is known. Ideally, a lot of this training data is needed, but this may be hard to come by. One of the aims of CMIH is to develop methods that can make do with very little data (an example is something called semi-supervised learning, which you can read about here) and still come up with accurate results.
Another problem is that the algorithms work like black boxes: they will give you an answer without telling you how they got there (see this article to find out more). In fact, the patterns an algorithm has spotted in the data may not even be discernible by a human. If such an algorithm suggests a potential diagnosis for a patient after analysing the available information, for example, then a clinician may not be able to figure out how it came up with the suggestion.
All these challenges push the boundaries of artificial intelligence more generally, not just in the healthcare setting.
Maths meets reality
Mathematicians can't solve the challenges involved all by themselves, which is why CMIH works with a number of partner organisations. These include the Cambridgeshire and Peterborough NHS Foundation Trust, pharmaceutical companies AstraZeneca and GlaxoSmithKline, companies that produce medical imaging equipment such as Canon and Siemens, and other organisations invested in health care, including the insurance company Aviva.
A recent meeting of CMIH mathematicians and representatives of some of those partner organisations, organised by the Newton Gateway to Mathematics in Cambridge, showed how the theoretical challenges are met with those arising from practical pressures.
One of these is about data. A vast pool of data — a data lake — is any algorithm's dream, but there are limits to how much information can be recorded about individual patients while ensuring they remain anonymous. This leaves room for algorithms to jump to wrong conclusions. As a simplified example, if a data set contains more young people with a specific disease than older ones, an algorithm may identify age as a risk factor for the disease. If, however, the data was collected in an area with a high proportion of young people, then age may simply be a red herring, masking the real risks at play.
In a slightly similar vein, mobility of AI tools is also a concern. If a tool has been designed on data from a particular country, or even a particular hospital, we need to be sure it can be reliably applied elsewhere. This can even be an issue when it comes to individual items of medical machinery: two scanners, even if produced by the same manufacturer, can give slightly different readings. A human eye can adjust to such differences quite easily, but they are enough to trip up even clever algorithms.
Ultimately all this feeds into the issue of trust. If clinicians are to use computerised tools, they need to be sure they work. This means that the tools, and the methods by which they have been tested, need to be transparent and explainable — not just to highly trained mathematicians, but also to lay people. It's something that mathematicians need to keep in mind right from the start when designing an algorithm.
Science fact not fiction
These challenges are already being addressed by CMIH researchers through various collaborations with the partner organisations. The main focus here are the most challenging public health problems — cancer, cardiovascular disease, and dementia. The aim of the Newton Gateway event was to check in on those research projects already underway and find more ways for mathematicians, clinicians and people from industry to collaborate.
It's important to note that the goal of the researchers is not science fiction — maths-based clinical tools already exist. One example is fractional flow reserved computed tomography (FFR-CT) which uses the equations of fluid dynamics to compute a 3D model of the arteries that lead to a person's heart. Another is a recent study which found that machine learning algorithms can outperform clinicians when it comes to identifying and classifying hip fractures. And, of course, mathematical modelling is playing a major role in tackling the COVID-19 pandemic.
There are many aspects of a doctor's work that machines will probably never be able to perform, and neither would we want them to. But tools such as those being developed by CMIH, if used correctly, will enable clinicians to focus on the tasks that only humans can do.
The image above shows a functional MRI image of the brain. It is taken from a Plos One article by Miller AH, Jones JF, Drake DF, Tian H, Unger ER, Pagnoni G, and reproduced here under CC BY-SA 4.0.