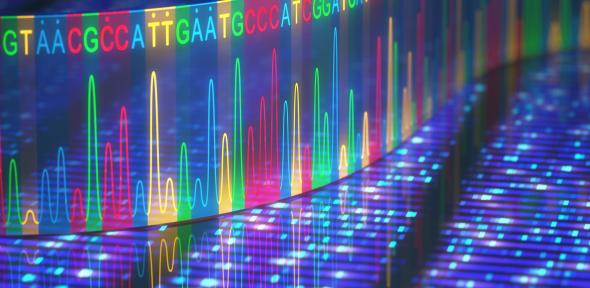
Last month the Statistical Laboratory welcomed a new Director: Professor of Statistical Science, Richard Samworth. The Laboratory was established seventy years ago in a "temporary hut" on Corn Exchange Street. Since then it has become a vital part of the Department of Pure Mathematics and Mathematical Statistics, moved into the Centre for Mathematical Sciences, and grown into a world-leading research centre in its field. What kind of work is being done at the Stats Lab and why is it important?
"The societal impact of statistics is enormous," says Samworth. "It gives us a basis for making evidence-based decisions. Should we put up interest rates? What should we do about climate change? Does the Higgs boson exist? These are questions we can try to address by collecting appropriate data and then performing careful statistical analyses. The economic impact is also very big indeed. If you imagine that statistical algorithms underpin driverless car technologies, or how we might try to improve London's transport network by collecting Oyster card data, and these sorts of things, there is huge economical potential from the use of statistical methods."
Big Data and random geometry
Data science will be a big growth area over the coming years. The demand from applications is so great, I don't see it diminishing. Richard Samworth
The Stats Lab covers four main areas: statistics, probability theory, mathematical finance, and operations research. All are motivated by the need to understand random phenomena. "In statistics a lot of our work is motivated by the modern phenomenon of Big Data: the fact that it's now possible to collect and store data on previously unimaginable scales," says Samworth. Whether it's medical data collected from individuals by smart watches, data from financial markets, or information gleaned by telescopes about the furthest reaches of the cosmos, the new wealth of data presents huge opportunities to answer questions about the world that were previously out of reach. But it also poses great challenges. "A lot of the algorithms that we know well and that work well in low-dimensional problems, or where you have a small number of data points, can either not be used at all or might perform very badly in this new setting." New algorithms are needed, then, and their suitability needs to be thoroughly tested.
"In probability [theory] many of the researchers [at the Statistical Laboratory] are motivated by questions to do with random walks, random surfaces, or random shapes more generally," says Samworth. For example, the fluctuations of stock prices over time might be modelled as a random walk: the motion of the price, whether it goes up or down at a given moment in time, and by how much, is so unpredictable, it might as well have been decided by the roll of a die or the flip of a coin. "There is a great interplay between randomness on the probability side and the geometry of the shapes that random processes form," says Samworth. "So people try to classify these different processes into different classes." (For an example of this kind of work, see this interview with former PhD student Ellen Powell.)
Seeing through the data jungle
Samworth's own work, for which he has recently been awarded the Adams Prize (jointly with Graham Cormode from the University of Warwick), provides examples of the kind of problems that come with very large data sets. Imagine you are a geneticist who wants to measure the expression levels of many thousands of genes and find out which genes are relevant for a particular disease, typically using only a small number of replications of your experiment. You are faced with a so-called variable selection problem. "This is a problem where you have many variables you may initially want to collect data on, and you try and ascertain whether you need [all these variables] in your model or not," says Samworth.
Over recent years there has been an explosion of work on variable selection problems and many different methods that work in different circumstances have been proposed. Together with former PhD student Rajen Shah (now a lecturer at the Stats Lab), Samworth proposed a way of employing existing methods more efficiently. "It's a very simple idea. Instead of applying a method once to an entire data set to see which variables are important, you apply it many, many different times to half of the observations at a time: you randomly choose half of the observations and you keep noting which variables would be selected if that was your full data set. Eventually you choose the variables that keep cropping up again and again on each of the subsamples." Samworth and Shah's method is general enough to work with any original base procedure and with any underlying data generating mechanism. And crucially, it allows statisticians to control the types of error they might encounter in a variable selection problem. (See the video below for another example of Samworth's work.)
Need help? Visit the Statistics Clinic!
Samworth and his 17 academic colleagues at the Stats Lab are experts in all things random and complex, but the same can't be asked from scientists in other fields: it's not unusual for a researcher from another area to get stuck on statistical question. To help out, Samworth set up a "stats clinic" at DPMMS about eight years ago (he was already working there at the time, but not as Director). "Once a fortnight everyone in the University can come and get help with their statistical problems," he explains. "It's been a very insightful [experience] for me. You'd expect to get a lot of researchers from the biological sciences, and we certainly do. But we also get people from all sorts of different domains that you wouldn't necessarily imagine, like linguists, historians, and musicologists. The variety of the questions they come with is very stimulating."
"It's also a great way for me to train my PhD students and postdocs. It takes real skill to distil the essence of someone's problem in a way that you can understand, and to then propose a solution. It's also really important to have the ability to communicate the advice in a way that's understandable to the practitioner you are talking to. That might mean recommending a rather different method [than you first had in mind], depending on the ability level of the person you are speaking to."
The future
And what about the future of the Statistical Laboratory and the field as a whole? "It's very difficult to predict what are going to be hot research topics in the long-term future," says Samworth. "But this year we are seeking to appoint a new Churchill Professor of Mathematics for Operational Research. That's partly because optimisation is crucial for a lot of modern statistical algorithms. [These algorithms] often involve many different variables and are highly complex, so we're looking for someone with expertise in that area."
"Data science will be another big growth area over the coming years. The demand from applications is just so great at the moment, I don't see it diminishing." With these prospects, it's clear that the Statistical Laboratory is never going to find itself in a temporary hut ever again.
For another example of work done at the Statistical Laboratory see Changing the way we communicate risk.