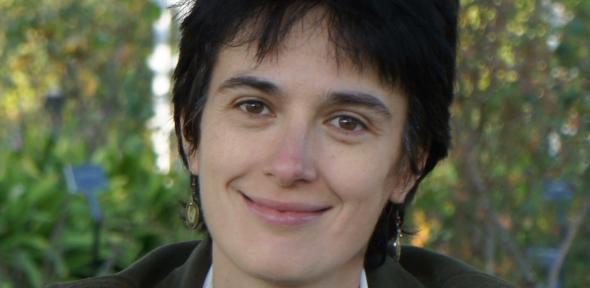
A recent partnership between a research group at the Department for Applied Mathematics and Theoretical Physics (DAMTP) and the NHS aims to help hospitals manage capacity during the COVID-19 pandemic and beyond.
Since 2018 Mihaela van der Schaar has been the John Humphrey Plummer Professor of AI, Machine Learning and Medicine at the University of Cambridge. Her role follows professorships at UCLA (ongoing) and Oxford, in addition to a fellowship at the Alan Turing Institute. Most recently, she created the Cambridge Centre for AI in Medicine.
The augmented doctor
I'm cautiously optimistic about the prospect of turning what we're doing today with COVID-19 into a broader framework for helping hospitals to manage their resources. Mihaela van der Schaar
Van der Schaar summarises her lab's work as "creating and applying cutting-edge machine learning methods that will drive a revolution in healthcare." Her vision is to develop machine learning tools that can provide clinicians and researchers alike with reliable support in decision making and actionable intelligence far surpassing the capabilities of frequently used statistical methods, or even other existing AI-driven techniques. Applications could range all the way from predicting mortality rates after organ transplant to personalising training courses for doctors. She and her team call this "the augmented MD."
At the centre of machine learning lie algorithms that can spot patterns within data (e.g. data sets relating to patients) and in response to these patterns return a particular output (e.g. whether a patient will need a particular form of treatment). Initially the algorithm's ability to output the correct answer may be low, which is why it is given examples of data sets for which the correct answer is known — so-called training data. While processing the training data, the algorithm will tune the values of parameters that have been built into it, until it gives the correct output with a high probability. Once this is the case, the algorithm can be used to reliably provide correct answers for live data. (You can find out more about machine learning in this article on Plus magazine.) It may seem like magic at first, but machine learning algorithms have now become so sophisticated they can be used in contexts where correct prediction is crucial, such as the medical settings van der Schaar's team is working on.
Due to the nature of their work, van der Schaar and her researchers collaborate extensively with doctors. Since doctors are among the intended end users of their models, this time spent together helps the researchers formulate medical problems that can be solved through application of machine learning and enhances their ability to conceptualise what answers to those problems might look like. Collaboration with doctors is also crucial since machine learning models are trained on patient datasets, and those datasets (even when depersonalised) are only ever provided with a high degree of caution. Among other things, van der Schaar's team have worked extensively to develop prognostication tools for breast cancer, cardiovascular disease, and cystic fibrosis. But their most recent collaboration is with the NHS and Public Health England, and relates to the ongoing COVID-19 pandemic.
The call to action
Van der Schaar's involvement with the fight against COVID-19 began in late March, when her research team and a number of collaborators published a perspective paper calling on governments and healthcare authorities to use proven AI and machine learning techniques and existing data to coordinate a response to COVID-19. Their particular focus was finding ways to alleviate the likely burden on social and healthcare infrastructure. "AI and machine learning," van der Schaar wrote, "can use data to make objective and informed recommendations, and can help ensure that scarce resources are allocated as efficiently as possible."
Among the proposals put forth by the team was the generation and provision of "accurate patient risk scores that will help clinical professionals decide who needs urgent treatment (and resources), and when." In essence, this would involve using state-of-the-art machine learning techniques to predict which patients would be most likely to require an ICU bed or ventilator, and aggregating these predictions to provide a hospital-level or regional picture of total demand for these resources over time. These predictions could be based on electronic health record data and information recorded when a patient first arrives in the hospital — including lab test results, age, gender, other medical conditions, risk factors, and time between onset and diagnosis of a disease.
The response
Almost immediately after the team published their perspective paper, van der Schaar and her team were contacted by Public Health England, who provided a set of depersonalised data on existing COVID-19 cases. They spent the next few days training models on this data, and within a week were able to create a proof-of-concept demonstrator showing that machine learning techniques can accurately predict how COVID-19 would impact resource needs (particularly for ventilators, ICU beds) at the individual patient level and the hospital level.
The short turnaround time was enabled by the inherent versatility of Cambridge Adjutorium, an in-house prognostication tool developed by van der Schaar and her researchers. Driven by a state-of-the art machine learning model, Cambridge Adjutorium was conceived a couple of years ago as a prognostication tool for cardiovascular disease, but, as van der Schaar explains, it was "created from the outset for use with a broad range of diseases and conditions." It has been validated for cystic fibrosis and breast cancer, and training the system on the COVID-19 patient dataset provided by Public Health England demonstrated that its predictive accuracy far surpasses existing and widely-used survival analysis techniques.
Building on their initial proof of concept, van der Schaar and her researchers continued to work with an ever-expanding set of data provided by Public Health England, while also entering discussions with NHS Digital regarding potential implementation of Cambridge Adjutorium in a real-world setting.
The partnership
The next few days involved extensive collaboration and ongoing development and feedback cycles between van der Schaar’s team of machine learning experts and the clinicians and data scientists of the NHS. As Dr Jem Rashbass, NHS Digital's executive director for master registries and data, explains, "the van der Schaar Lab showed it was possible to do capacity planning for COVID-19 patients. We recognised that there was an opportunity to deploy this as a service and deliver a real data-driven planning tool to hospitals."
Within roughly a fortnight, the Cambridge Adjutorium COVID-19 proof of concept had evolved into a tool that could be deployed at NHS Trust hospitals. "Obviously a rollout at scale on day 1 wouldn't have been possible or helpful, so the number of hospitals would need to be limited before considering scaling up," says van der Schaar.
At the end of April, the team announced their partnership with NHS Digital to adapt Cambridge Adjutorium to power NHS Digital’s Capacity Planning and Analysis System (CPAS), which would initially be trialled at four "alpha sites" in England in order to demonstrate its viability for broader usage. The trial is expected to run until it can be reliably scaled up over the coming weeks.
Van der Schaar and her team are grateful to be able to play a role in responding to the current pandemic, while also serving their mission of applying machine learning methods to advance healthcare.
"Helping clinicians save lives is at the very core of our work," she says. "We're thrilled to be able to work with NHS Digital and Public Health England on this unique and pioneering project."
The future
For van der Schaar, the lab's partnership with the NHS represents the wider change she hopes to see in healthcare.
"I'm cautiously optimistic about the prospect of turning what we're doing today with COVID-19 into a broader framework for helping hospitals to manage their resources," she says. "If projects like this can yield deeper collaboration and lay the foundation for more robust digital infrastructure for healthcare, we can come out the other side of this crisis even stronger."